Svetlana Borovkova: Fairness in AI Part II
Svetlana Borovkova: Fairness in AI Part II
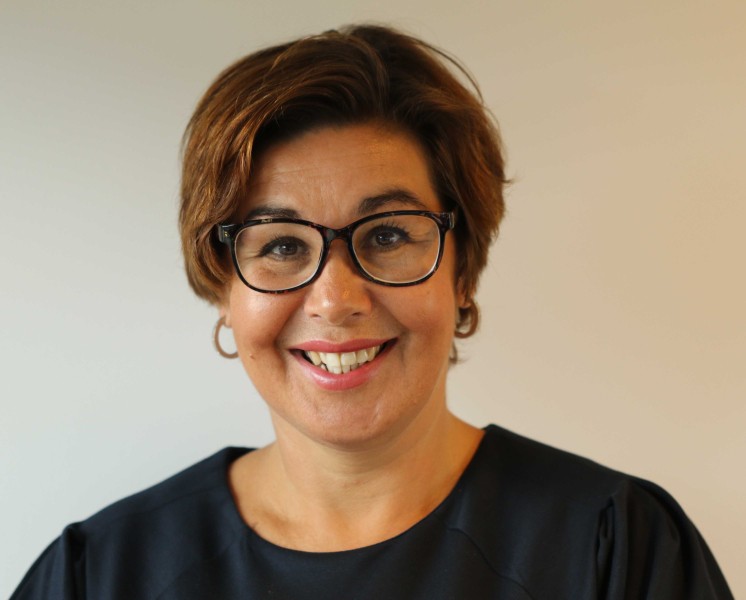
By Svetlana Borovkova, Head of Quant Modelling at Probability & Partners
In my previous column, I discussed the bias in machine learning algorithms (i.e., (un)favourable treatment of individuals based on their race, gender or other protected attributes) and pointed out that such bias can be damaging for financial institutions, especially in light of the current regulation.
Today, I would like to give some practical insights into how the bias in ML algorithms can be measured and where in your modelling process it can be eliminated.
Measurement of the bias is the first important step: an algorithm can be ‘very’ biased (think of the Apple/GS credit card example from my last column) or a ‘little bit’ biased (and a small bias could be something you can live with). There are three ‘forma’ definitions of the model’s fairness: Independence, Separation and Sufficiency, and hence, three ways of measuring bias.
Independence and Separation
Independence is the ‘crudest’ fairness definition and it strives for equal outcomes for favourable and less favourable groups. For example, this means that men and women should have the same overall chance of getting a credit. This definition is very simple and easy to understand. However, there could be a lot of heterogeneity between the groups of men and women, and so this definition can be too crude to appropriately address the bias.
A more subtle definition is that of Separation. To explain this definition, imagine you have a sample of men and women in your dataset, for whom you know whether they defaulted on their credit in the past. You have built a ML model which predicts whether someone will or will not default (and your credit issuance decision will be based on this prediction), and you apply this model to those individuals.
Separation means that, if you consider only those people who defaulted, the chance that you model also predicts them to default should be the same for men and for women. The same, by the way, should hold for those who did not default. In mathematical terms, this means that true and false positive prediction rates should be the same for men and for women.
Sufficiency
The last definition, quite similar to Separation, is Sufficiency, only here we swap what we predict and what really happened: consider only those people who we predicted to default. Among those, the proportion who really defaulted should be again the same for men and for women (and the same should hold for those who we predicted to default but who did not default in reality).
The magnitude of your model’s bias is the discrepancy between those probabilities (in either of the three definitions) that should be equal. Usually we use the so-called four-fifth rule: the difference between these probabilities should be not more than 20%, or we deem the model significantly biased.
Some solutions
Which of the three ways the bias is measured is usually left up to the model builder. Suppose the bias has been measured and it turns out to be too high. What now? Well, there are also three points in your model where it can be improved in that respect. First thing you can do is to modify the data used to train the ML model.
This can be done via the so-called ‘massaging’ (swapping some of the outcomes between the advantaged and disadvantaged groups), re-weighting or changing features to increase fairness. We call it bias mitigation in pre-processing stage.
Another solution is to modify ML algorithm itself, so that it becomes less biased. This can be difficult and costly, as most model builders rely on ready-made algorithms which are not easy to change. Finally, you can also change the model outcomes to increase fairness – this mitigates bias in the post-processing stage.
Any bias mitigation algorithm would typically decrease the performance of the ML model. So the bias mitigation is a balancing act between improving fairness while not sacrificing too much of the performance. However, this balance is easily achieved: the modern bias mitigation techniques do not require too much of the performance loss, while significantly improving fairness of your ML model.
You should worry
As an asset manager, should you worry about fairness of ML algorithms? Definitely yes, as AI and ML are increasingly applied in your industry, with applications ranging from investment robo-advisory to asset choice and allocation. All these applications are prone to bias in some degree, just as human investment decisions are prone to a multitude of behavioural biases (which can be amplified by ML algorithms).
There is another channel of exposure to harmful ML biases: investing in credit portfolios where credits are issued on the basis of biased algorithms, or in firms that potentially discriminate against certain groups due to biases present in their decision making (or decision-aiding) AI tools.
Probability & Partners is a Risk Advisory Firm offering integrated risk management and quantitative modelling solutions to the financial sector and data-driven enterprises.