Svetlana Borovkova: Choosing the data provider for your sentiment strategies
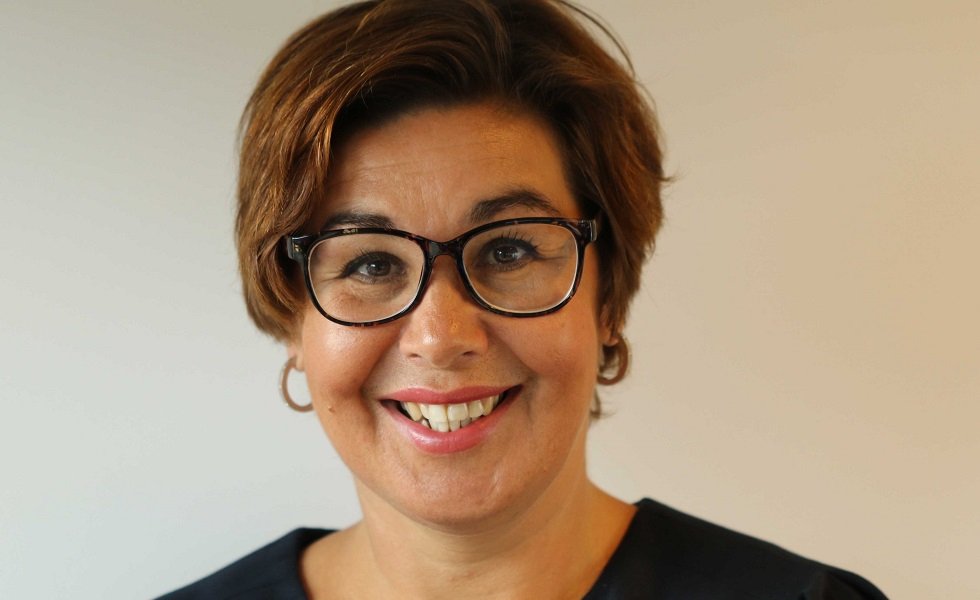
By Svetlana Borovkova, Head of Quant Modelling at Probability & Partners
Last April, I wrote two columns about using news and social media sentiment in investing. I argued that financial markets are largely driven by the perception of market participants and that media sentiment reflects this perception almost instantaneously.
Moreover, official news contains fresh and timely information that is rapidly incorporated into asset prices. News sentiment therefore provides an investor with an early signal to act upon.
At Probability & Partners, we see more and more asset managers willing to incorporate sentiment-based signals into their investment strategies.
However, developing sentiment-based investment strategies, or incorporating sentiment in your existing investment process, is a non-trivial and multi-step task.
Steps towards incorporating sentiment in the current investment process
First, one must define the framework in which sentiment signals will be used and their purpose (for instance, alpha generation or risk reduction).
Then, news and/or social media content must be collected and put through Natural Language Processing machinery in order to quantify the sentiment and other characteristics.
Next, it is necessary to process the sentiment numbers by, for instance, de-noising and aggregating them through time and over the investment universe.
Finally, the resulting sentiment indicators should be included in an appropriate way into quantitative or blended investment strategies, which ideally should be back-tested on historical data.
Obtaining the necessary sentiment data
In this column, I would like to address the second step in the above process: how does one obtain the necessary sentiment data?
In order to get the sentiment investing signals, we first need to ‘crawl’ news and social media sites on the Internet, collecting content that is relevant for our asset universe.
Then this content needs to be processed by sophisticated Natural Language Processing (NLP) algorithms to discern its relevance for our portfolio and, most importantly, to determine its sentiment, the ‘mood’, of the collected news items and social media messages.
Given the volume of material, this is effectively an impossible task. Even for a seasoned quantitative analytics team.
Fortunately, there are plenty of providers of sentiment data for financial (and other) applications. Fundamentally, they all do the same thing: their web crawlers collect the content from the Internet and their NLP algorithms process it for sentiment and other quantitative characteristics.
So, given all these providers seek to do the same thing, how should one set about choosing an appropriate sentiment data provider? What distinguishes them from each other?
Choosing an appropriate sentiment data provider
Let me first point out that it is not their NLP capabilities – these are typically similar among sentiment data providers – but a multitude of other characteristics.
First, it is the type of content their algorithms analyse. This content can be either official news or social media (WSJ, SeekingAlpha, MarketWatch, Benzinga and others) or both. For institutional investors, official news typically provides more reliable signals. It is important to understand whether the data provider uses public (free) or paid-for news sources. The latter should be preferred.
For individual investors and hedge fund-like strategies, social media could be preferred. Here it is important to ask whether Twitter content is included or not. And if so, it is important to consider which steps are taken to filter out relevant content and make sure only tweets from ‘reliable’ or ‘influential’ sources are included.
The second criterion is the asset universe for which the sentiment is provided. How many stocks are included and from which markets? Is sentiment for commodities and currencies available? Are there also sentiment signals for stock markets and indices?
Next criterion is the type of output. What quantitative features (other than sentiment) are available? Examples of such characteristics would be the volume of content, ‘buzz’ surrounding a certain stock, novelty and relevance of news and messages. All these characteristics can also have a significant influence on the price movements and should be included.
Other questions to ask would be: with which frequency are the sentiment signals updated? Is it daily or intra-day? What is the length of the provider’s history, for back-testing the strategies? What languages are included in their NLP engine? How are the data accessed? Are they easy to access and process or are additional processing steps required?
All these things should be evaluated in relationship to your investment philosophy and strategies. For example, if your portfolio predominantly contains European stocks, then you should probably look for a sentiment data provider that also incorporates multiple European languages and not just English language news and social media content.
Finally, there is the question of cost. Sentiment data are typically quite expensive and the cost-benefit analysis must be done. At Probability, we have developed a framework for valuing of alternative data. This will covered by one of my future columns.
Meanwhile, if you are thinking of using sentiment in your investment strategies and are baffled by all the different data offerings, get in touch and we at Probability will help you decide which sentiment offering best suits your investment strategies.
Probability & Partners is a Risk Advisory Firm offering integrated risk management and quantitative modelling solutions to the financial sector and data-driven enterprises.