Svetlana Borovkova: Machine learning in finance – now and looking ahead
Svetlana Borovkova: Machine learning in finance – now and looking ahead
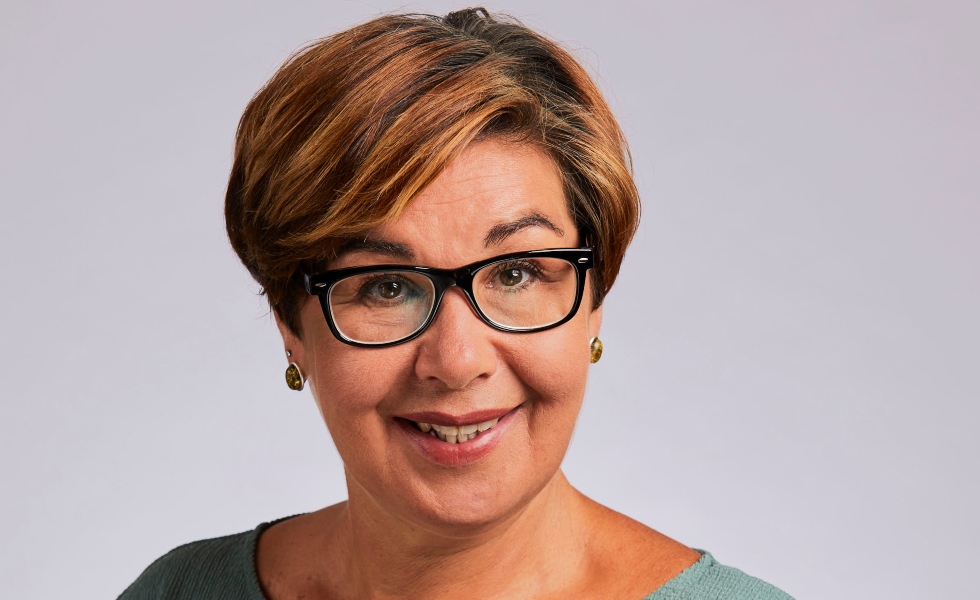
By Svetlana Borovkova, Head of Quant Modelling at Probability & Partners
‘AI wave is coming’: this catchy phrase opens the recent SAS and GARP survey on Artificial Intelligence in Banking & Risk. The phrase perfectly summarizes the arrival of machine learning in financial services.
What is the difference between AI and machine learning? AI can refer to anything from a computer program playing chess to a voice recognition system such as Amazon’s Alexa, which interprets and responds to speech.
AI technology can be broadly classified into three levels:
- Narrow AI. These are systems designed to perform a single specific (albeit complex) task. Examples are IBM’s Deep Blue, which beat the World’s leading chess grand master Garry Kasparov in 1996, or Google’s Deep Mind Alpha Go, that beat the World’s Go champion Lee Sedol in 2016.
- Artificial General Intelligence (AGI). These are systems that are aimed to achieve human-level intelligence. Amazon’s Alexa is an example of progress in this direction.
- Super Intelligent AI. According to the definition of super intelligence, it is an intellect that is much higher than that of the best human brains in practically every field, including scientific creativity, general wisdom, and social skills. This level of AI remains science fiction for now and probably decades more - if not longer.
What about machine learning (ML)? Machine learning refers to algorithms that provide knowledge to computers through data, observations and interacting with the World. In other words, machine learning is the science of getting computers to act without being explicitly programmed. We can also say that machine learning is what powers artificial intelligence.
ML in banks: from credit to fraud
Potential applications of machine learning can be found in all areas of finance: from banking and insurance to trading and asset management. The first financial institutions to embrace ML were algorithmic trading firms, who developed machine learning algorithms for trading (typically at high frequency). At the moment, several well-developed ML applications can be found in banks.
Machine learning classification algorithms are excellent for assessing creditworthiness of loan applicants and for monitoring existing loans – I discussed such credit-related applications of machine learning in my Financial Investigator column of November 23, 2021. However, in this area, the main barrier to using ML in banks’ daily operations is lack of regulatory acceptance.
Another area, where ML is already successfully used, is transaction monitoring, in which suspicious transactions (fraud or money laundering) are automatically flagged. Here, ML classification algorithms are trained to detect ‘abnormal’ transactions based on numerous characteristics, such as: transaction size, sending and receiving parties and countries, or the presence of similar (or very different) recent transactions. Given the enormous volume of transactions, automation of this process using machine learning is extremely useful, saving a lot of time and money
Several large banks (including some in the Netherlands) are already using ML for this task. However, a problem can be the lack of the so-called labelled, or tagged data: a large historical set of transactions known to be fraudulent or related to money laundering. Such data, on the basis of which a machine learning algorithm can be trained, is rarely available, and one must resort to the so-called unsupervised learning: a class of machine learning algorithms which aim to classify unlabelled data into groups, or clusters.
After training such algorithms on unlabelled transactions, one can hope that one of the resulting clusters will correspond to the ‘suspicious’ transactions. Such algorithms are only partially successful, and more work is needed to improve their detection power – which is currently an ongoing effort by researchers as well as financial fraud/AML experts.
Other ML applications: derivatives, market risk and quant investing
Recently, there have been many academic and practitioners’ studies on the use of machine learning for trading and hedging of derivatives, such as options. Some derivatives desks of large banks are already experimenting with such machine learning pricing and hedging models, alongside traditional ones. Here, a machine learning algorithm ‘learns’ what an option price (on a particular underlying asset) should be, given the option’s characteristics such as strike and maturity, and current volatility level. This can be thought of as an approximation algorithm, replacing, for example, the well-known Black-Scholes formula.
Another ML derivatives application – hedging – can be seen as the combination of an ‘action’ (for instance, delta-hedging) and a ‘reward’ (hedging cost), which puts this problem into the framework of the so-called reinforcement learning. Hedging derivatives using reinforcement learning can be very successful, and even more successful than traditional models, as we show in the recent Probability & Partners’ white paper by Alexandru Giurca and myself.
A very recent and exciting application of machine learning is in market risk. This application is so ingenious and potentially so useful that I will devote a future column to it.
Another topic for a future column is the use of machine learning in quantitative investment strategies. In all, you can see we are excited at Probability & Partners about machine learning finding its way into finance. And I hope you will get just as excited about it as I am!
Probability & Partners is a Risk Advisory Firm offering integrated risk management and quantitative modelling solutions to the financial sector and data-driven enterprises.