Nuklai: Data as an asset for AI innovation
Nuklai: Data as an asset for AI innovation
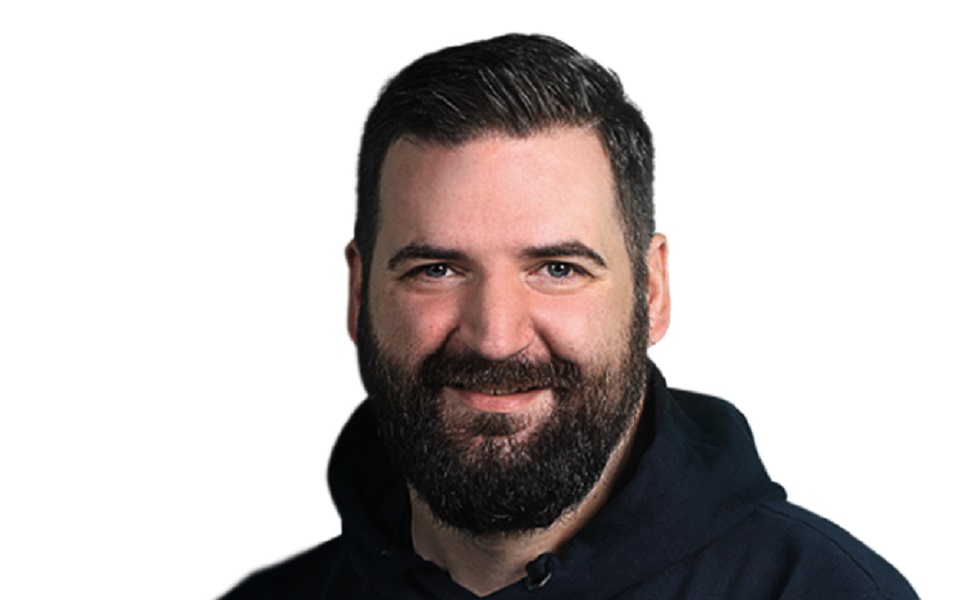
Over 67% of the global population is online. This gives businesses easy access to an invaluable asset: data. Data is more valuable in an AI-driven economy as it is the oxygen for AI-driven applications.
By Matthijs de Vries, Founder & CEO, Nuklai
Data is the hero of the AI world. Without it, AI algorithms could not understand or predict the nuances of human interactions. Nor could they deliver the personalized solutions that are the industry’s future. The synergy between data and AI has an amazing impact. In business, datadriven AI can enhance operational efficiency, process, and product innovation. AI can lower cycle times and improve maintenance schedules. It can also strengthen security and reduce the carbon emissions of companies.
According to the EU’s European Institute of Innovation and Technology (EIT)1 , data and AI are the most significant drivers of Europe’s current and future economic growth. So, data is a pillar of a sustainable economy since it is a determinant of enterprise value creation. It is also a vital metric for societal well-being.
Data opportunities in a world of AI-driven applications
Data is an asset class. First, for established businesses with large historical and real-time data reserves. For instance, Bloomberg monetizes its data via BloombergGPT, an LLM (large language model) for the finance sector. But this model has over 50 billion parameters. Businesses need access to significant amounts of accurate and trustworthy data to train such LLMs. They also need access to powerful GPUs (graphics processing units). Robust compute resources keep these models learning and getting better at insights. To this end, organizations seeking such an opportunity must set aside significant data and AI innovation budgets. An alternative is mining value from data via data ecosystems that provide seamless integrations to data standardization processes, compute integrations, and LLM developers. These data and AI solutions are affordable and accessible to most businesses.
Second, some businesses host invaluable but idle datasets. Twitter and Reddit, for instance, have vast humangenerated content. They are turning it into monetizable assets via data APIs (application programming interfaces) and access subscriptions. Now, AI companies can train their models using their content, generating new value from this data.
Businesses need access to significant amounts of accurate and trustworthy data.
Third, many small and medium businesses also hold unique proprietary data. For instance, let’s review the data opportunities available to a retailer. Many e-commerce databases hold years of customer reviews in text and image format. Some businesses also store years’ worth of audio recordings of customer service interactions. This data can provide priceless insights into their buyers’ preferences. Such businesses can leverage LLM tools that mine insights and trends from their data. AI will identify trends and make accurate predictions impacting a retailer’s bottom line. Another option for a retailer is building a custom AI application using its data and AI toolkits. After that, they can monetize their tools to generate revenue for their business.
Data also opens doors for pick-and-shovel platforms that reshape data into refined gold or smart data. A good example is the development tool builder or the data assets marketplace. These are platforms that connect data assets to AI infra. They standardize, generalize, and then extract value from data. So, they are consumers of data and producers of rich datasets.
Then again, data can create value for ecosystems that enhance data privacy and compliance for other businesses. These platforms ensure that data marketplaces place consent over usage. They do so by streamlining access to data and eliminating third-party data handlers. A middle-manfree data ecosystem will also ensure fair value distribution amongst all stakeholders.
AI innovation has brought about an onslaught of hallucinated content, deep fakes, and spam content. This is an opportunity for innovators who can enhance data’s trustworthiness and provenance. These businesses will also reap value data and AI. Then, plug-and-play LLMs like ChatGPT can enhance business operations and foster innovations. But general-purpose LLMs lack domain-specific expertise, so they underperform in unique contexts.
That said, businesses can fine-tune LLMs with sectoror organization-specific data. Customizable LLMs provide deeper context, expertise, and accuracy in specific tasks. They are, for instance, popular as medical diagnosis, legal research, or algorithmic trading tools. Businesses can also blend low- or no-code tools with datasets from data platforms. For example, they can leverage data marketplaces to access diverse niche datasets for LLM innovations. Then, savvy businesses with unique data can also gain new insights via collaboration. They can, for instance, partner with diverse subcommunities or competitors via data consortiums.
Enterprises should not just collect data. They should clean, transform, and standardize it to turn it into valuable assets.
Moreover, data consortium technology supports the development of LLMs. Its protocols also protect the autonomy and control of proprietary datasets. The best collaborative data platforms integrate algorithms into data at its source. As a result, business data never leaves its storage. So, its holders stay compliant with privacy regulations. These protocols also give data consortium members autonomy over proprietary data.
Last, there are opportunities in the hardware semiconductor, infrastructure, and developer tool layers. These sectors offer capitalintensive, super-competitive, and concentrated data opportunities. These layers have created value for large corporations like Google and Nvidia. For this reason, there is little to no space here for underfunded startups.
How businesses can reap value from data in a world of AI
Most businesses collect large amounts of data, but it is often not labelled. Labelling data is a time- and resourceintensive exercise. It may involve the complexity of NLP (natural language processing) and object recognition technology. Businesses often collect and store their data for years. However, time may render such data useless. That means other businesses use unreliable collection methods. They cannot leverage it as an asset as they are unsure of its accuracy.
The other challenge hampering the value of data as an asset is its lack of diversity. Data from a single source lacks variations. Variance ensures that data insights represent a true target population. To this end, data preprocessing is vital in its valuation. Enterprises should not just collect data. They should clean, transform, and standardize it to turn it into valuable assets in the age of AI.
High-quality and enriched data provides accurate and reliable insights. It lowers bias and errors in the AI training process. As the saying goes, in data, quality scales better than size. To take part in the data and AI revolution, businesses must collect and store high-quality data.
SUMMARY Data is the new asset class in the world of AI-driven applications. Enterprise opportunities for data include:
|