Probability & Partners: AI innovation with accountability
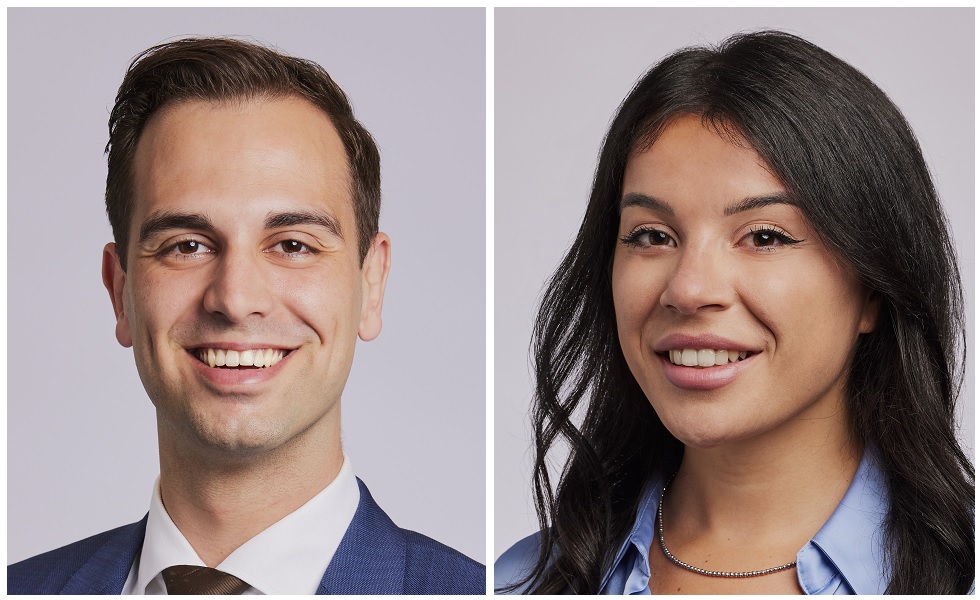
By Erik Kooistra, Senior Risk Management Consultant, and Chiara Trovarelli, Financial Risk Management Consultant, both working at Probability & Partners
Machine learning (ML) has revolutionized risk management. From predicting credit defaults to detecting market anomalies, ML offers unparalleled accuracy and efficiency. It allows organizations to leverage vast datasets, identifying trends and correlations that were once invisible or too complex to model.
The promise of ML lies not only in its computational power, but also in its ability to continuously evolve through self-learning mechanisms. However, despite its groundbreaking capabilities, ML faces a critical challenge: interpretability. In today’s heavily regulated financial landscape, models that can’t be clearly understood or explained pose significant risks, not just for operational performance but also for regulatory compliance and strategic business decisions.
Lack of transparency
The problem is clear: although ML models deliver high performance and efficiency, they are often perceived as ‘black boxes’. This lack of transparency leads to major issues when implementing these models in highly regulated environments. Financial institutions are left grappling with the challenge of trusting predictions they cannot explain. This opacity raises concerns related to compliance in critical domains such as accountability, fairness and data protection - particularly under regulatory frameworks like CRR3, the AI Act, and GDPR. Regulatory bodies are increasingly demanding not just robust performance, but also clear, justifiable explanations behind automated decisions.
Bridging the gap
The solution to this challenge is Explainable AI (XAI). XAI bridges the gap between innovation and transparency, making complex model outputs understandable for both technical and non-technical stakeholders. It enables users to track how models make decisions, for both global datasets and local data points, uncovering insights into data influences and patterns. In an industry where accountability is crucial, XAI not only safeguards compliance, but also fortifies stakeholder confidence by demonstrating how and why predictions are made.
Our approach at Probability & Partners emphasizes human-centered AI that meets compliance expectations while fostering ethical innovation. Through pioneering methods like SHAP, LIME, and PDP, XAI not only unveils the inner workings of ML models but also ensures alignment with global standards of transparency and fairness. By integrating XAI into our framework, we bridge the gap between technical insight and practical application, allowing institutions to build more trustworthy systems.
Development, validation, and model use
XAI proves invaluable across the entire model lifecycle for ML models, but particularly in three critical phases: development, validation, and model use. During development, it helps identify feature importance, detect biases, and enhance transparency. In the validation phase, XAI supports detecting non-linear relationships and maintaining consistency across datasets, while also clarifying validation outcomes for non-technical audiences. During model use, it provides ongoing performance monitoring and decision transparency, crucial for maintaining stakeholder trust. XAI's continuous monitoring capabilities ensure that model predictions remain accurate and reliable, even as data patterns shift over time.
An example of key application frameworks that leverage XAI in Model Risk Management can be as follows:
- For model development: Apply the same XAI technique (e.g., SHAP) to both interpretable models (like LOG) and complex black-box models (like GBM, XGB) to compare their behaviour. This helps determine which model structure offers the best balance between predictive reliability and explainability.
- For model validation: Use multiple XAI techniques (e.g., SHAP, LIME, PDP, ICE) on a single model to assess consistency, feature impact, and error patterns (Type I & II). This ensures the model behaves as intended and is trustworthy before deployment.
Both frameworks provide valuable strategies for integrating XAI into MRM. The first framework helps select the best-performing model, while the second ensures that a chosen model is well understood and trustworthy. As organizations continue to rely on AI-driven insights, implementing these frameworks can enhance transparency, accountability, and regulatory compliance.
Performance meets transparency
As financial institutions continue to embrace machine learning, the demand for explainability will only increase. XAI is not just an optional enhancement, it’s an essential element of responsible innovation. By integrating XAI into our risk management practices, we ensure that performance and transparency go hand in hand, meeting both regulatory requirements and business needs. The journey toward fully explainable AI might still be evolving, but each step forward enhances the harmony between innovation and accountability.